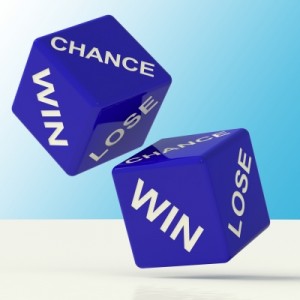
As the word indicates subjective Bayesianism is subjective. For that reason there are no means in subjective Bayesianism to compare the data from two different persons in an absolute way. The only thing you can compare is to what degree they improve or get worse.
For example: take person A who quantifies the quality of his life on a 1 (worst) to 10 (best) scale as an 8. Person B gives himself a 6. On face value the quality of life of person A is higher than that of person B. But this is not so for we don’t know whether this is really true. Person B might be much more critical than person A and if there were a celestial viewpoint of perfect objectivity allowing us to look inside the heads of both person A and B, it might turn out that objectively person B has a higher quality of life even though he only scores his life with a 6 rather than the 8 of person A. Of course there is no such viewpoint and even thinking about some way to overcome this limitation is fruitless. It is much better to learn to live it and use it.
Thus, if subsequently both persons practise NLP and both report an improvement of the quality of their lives, e.g. person A goes to 9 instead of 8 and person B reports an 8 instead of a 6, we cannot say which person has experienced the most improvement. The only thing we can conclude is that both have improved according to their own subjective criteria.
This is what we measure in the proto-scientific research, with one caveat: by using subjective Bayesian statistics rather than the subjectively reported values at face value, we are raising the bar for what it means to have an improvement in life quality measurement. In most data sets you can see what people subjectively reported and what subjective Bayesian statistics foresee as more likely results. In nearly all cases you’ll see that the subjective Bayesian statistics end up with lower values than those recorded by the persons involved. In our experience the former turns out to be much more reliable in relation to the future of these people than their own subjective reporting.
How much do you want to bet?
This is the really hard part of objective Bayesian statistics. We don’t want you to accept of reject the results of subjective Bayesian research. That would go against the grain of subjective Bayesianism. Instead, you have to realize that the data set for you is just another piece of information. Each one of use, including you, has to make up his own mind and come up with his own probability estimations . So we would never ask you to blindly accept or reject the outcome of any proto-scientific research we do. What we do want you to do is to follow subjective Bayesian procedure yourself in order to come up with your own probability estimations that you are willing to bet on.
The subjective Bayesian procedure involves three stages:
-
Find your personal and subjective probability estimation by considering which bets you would take and which bets you wouldn’t.
-
Check to see whether your estimations are rational in the sense that if you would bet on them they won’t involve a sure loss contract so you would always lose.
-
Update your personal and subjective probabilities with Bayes’ Rule whenever you get new relevant information about the event in question.
When you apply these three stages to NLP, we want you, before you look at the data sets in detail, first form an opinion on how likely you think that the topic researched would result in a positive event. For instance, if you think about NLP in general, you can come up with the probability that NLP would considerably improve people’s lives in the long run. As long as your estimation is somewhere between 1% and 99% you are pretty much guaranteed to be rational so you can go on into and through stage two very quickly.
In stage three you look at the relevant data sets in detail. Based upon all this new information you update your own probability estimation. You can do that with Bayes’ Rule or heuristically by coming up with a new number without the rigor of Bayes’ Rule. With each data set comes a proposal for a bet allowing you in principle to win up to 9800 euro. If your pro ability estimation is lower than the one the proposed bet is based upon (basically the result of the proto-scientific research), then we invite you wholeheartedly to enter into a bet with us on the proposed odds.
If you are right, you will make money. Depending on how far off your estimation is with ours, it might be a great deal of money. If we are right, nobody wins or loses any money. If we aren’t exactly right, (which we think is highly probable) you either win or lose a small amount. In other words there is no reason whatsoever not to bet against us if you disagree with the results of the proto-scientific research. You risk next to nothing and might win a big prize.
Or to put the argument upside down: if you refrain from betting against us, your true probability estimation is at least as high as ours, if not higher. If you happen to be a scientist this would be your true scientific opinion. We would love to hear from you if you refrain from betting against us as a scientist as this would elevate our proto-scientific research. As far as this, a scientist agrees with us. The best case would be of course that such a scientist would not ply agree with us but would also replicate the research and publish about it. That would truly be wonderful.
Complete freedom
Besides the data sets you have complete freedom in subjective Bayesian statistics to take into account whatever you want to take into account. For instance it is good to realize that we are probably working with a preselected group of people who have a positive bias towards NLP. How much this matters we don’t know, but it is something that you might want to take into account before accepting any bets. Of course such concerns don’t matter that much when you want to apply NLP to a broader group. If it turns out that a positive bias is of importance one comes up with a test to select people who are inclined to such a positive bias and teach them NLP.
Biases
As with all statistics, it is good to keep a couple of biases in mind when interpreting data. The following two biases are especially important within the field of NLP:
1) Selection bias.
The people who we work with are not an aselect group of people. In fact they have gone a rather extensive selection process before we work with them. Part of the reasons behind the high success rates is this selection process. Personally, we find nothing wrong with preselection as long as it is clear that a selection process is taking place. Unlike bad statistics Bayesian statistics works aswell with selection as with random groups. The selection process is another piece of all the information that one keeps in mind when doing Bayesian statistics.
2) Survivor bias.
Survivor bias is especially interesting for the field of NLP. Many NLP practitioners think that it makes sense to model experts to capture their skills that made them experts. It seems to make sense when you put it like that. Unfortunately, it overlooks survivor bias. The experts might not be experts at all and their good luck might be the only reason they are now considered expert. So rather than modelling their expertise a NLP practitioner might in fact just model their good luck. In the data you see here you see many NLP Master Practitioners. When you are interpreting data for them, please bear in mind that they are suvivors and more likely to do well. Currently two third of all people participating in an NLP Practitioner course go on to become a NLP Master Practitioner. So one third doesn’t survive the NLP Practitioner training to say it statistically. There are many reason why people stop doing courses. For many of the coachees the reason is that their problems are solved. For many of the NLP Practitioners who don’t become a NLP Master Practitioner the first course has already brought them everything they wanted to get out of a NLP training. For some though they reason that NLP is not for them. So, please keep these biases in mind when looking at the data.
2 thoughts on “Interpretation”